AI Communication and Technology
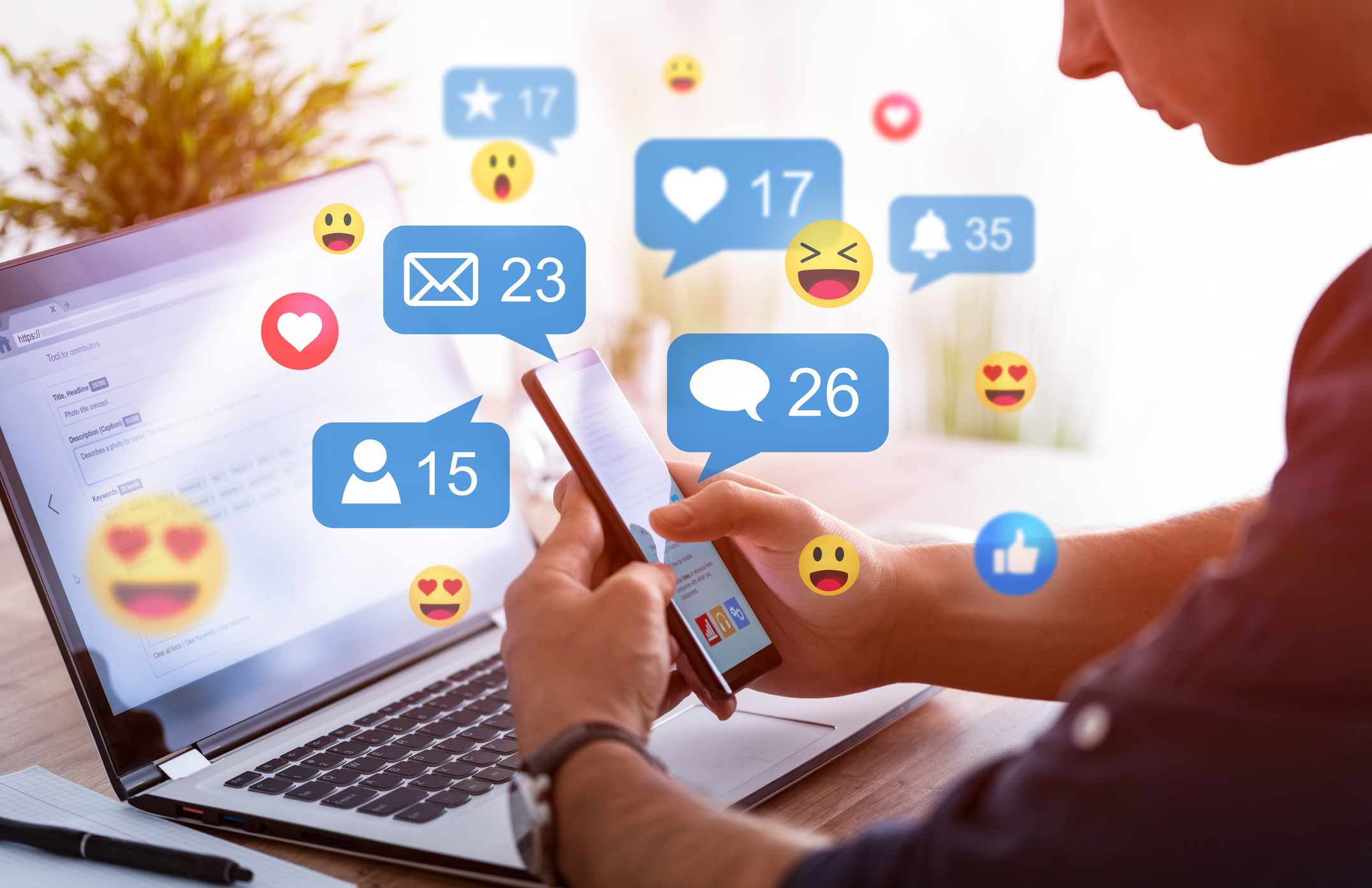
Using Artificial Intelligence to Analyze Consumer Sentiment
Many brands incorporate gender-related or political topics into their social media campaigns to stimulate conversation and engagement. However, consumer opinions are volatile, and a controversial campaign risks backlash. By using artificial intelligence (AI) to automate the analysis of consumer responses to social campaigns, brands can better understand consumer values and behaviors faster, more efficiently, and more effectively.
Researchers at the University of Florida College of Journalism and Communications wanted to better understand what type of algorithms worked best to interpret and classify user comments. Advertising Associate Professor in Artificial Intelligence Yang Feng and Advertising Associate Professor Huan Chen used the #LikeAGirl social media campaign by Always feminine hygiene to study which algorithms were more effective at analyzing comments on this campaign. Available on YouTube, the #LikeAGirl campaign garnered more than 40,000 consumer comments.
For the study, the researchers compared four traditional, supervised algorithms — a machine-learning method in which models are trained using labeled data — to deep learning, cloud-based AI models from Amazon and Google. This comparison helped to show how each algorithm performed and shed light on consumer insight.
The study started with a human-based content analysis of comments on the #LikeAGirl campaign. The gist of the campaign is to change the negative cultural meanings attached to the term “like a girl” to boost girls’ confidence.
The researchers wanted to focus on the ad sentiment of each commenter instead of the sentiments of the words used in the comments. According to the scholars, “… a commenter may be a strong supporter of a campaign and criticize other commenters who oppose the stance of the campaign. As a result, many of the words contained in the comment may be negative, but the commenter’s ad sentiment should be coded as positive. On the other hand, if a commenter detests the stance of a campaign and uses sarcasm to attack it, words contained in the comment may be positive, but the commenter’s ad sentiment should be coded as negative. In both cases, we need advanced techniques, such as supervised machine-learning algorithms, to understand the context of consumer comments.”
After watching the ads on YouTube, three human coders classified comments into four categories: 1) negative sentiment toward the campaign; 2) neutral sentiment toward the campaign; 3) positive sentiment toward the campaign; and 4) irrelevant sentiment. Then, researchers used different AI models to further examine the comments.
The study found that proprietary, deep-learning models from Google and Amazon had relative advantages over traditional machine-learning algorithms. For example, traditional algorithms may not achieve the high performances of proprietary deep-learning models, and training traditional supervised algorithms from scratch requires intensive coding, whereas using pretrained models from Google and Amazon requires limited coding.
The researchers also found differences in performances between Google’s and Amazon’s models. Results from Google’s models were not as consistent as those from Amazon, but Google’s was more transparent. Overall, they found that Amazon’s model was the most efficient AI tool, achieving satisfactory learning results and using the shortest processing time to generate reliable results.
This research also showed how to combine different types of algorithms to retrieve consumer insights and better understand consumer behavior.
Future research would do well to include a larger samples size and examine other social issue campaigns.
The original research paper, “Leveraging Artificial Intelligence to Analyze Consumer Sentiments within Their Context: A Case Study of Always #LikeAGirl Campaign,” appeared in the Journal of Interactive Advertising (October 2022).
Authors: Yang Feng, Huan Chen
This summary was written by Marie Morganelli, Ph.D.
Posted: October 31, 2022
Tagged as: AIatUF, Artificial Intelligence, Huan Chen, Social Media, Yang Feng, YouTube